- Mastering Observability
- Posts
- Putting It All Together: Building a Comprehensive, Cost-Effective Observability Strategy
Putting It All Together: Building a Comprehensive, Cost-Effective Observability Strategy
Beyond the Numbers Blog Series (5/5)
ObservCrew, what will you learn?
Intro
Welcome back, ObservCrew; to the grand finale of our "Beyond the Numbers" series. We've been on quite a journey, haven't we? From tackling the Observability cost conundrum to exploring open-source solutions, we've covered a lot of ground. Now, it's time to tie it all together and build a strategy that'll make your observability comprehensive and cost-effective.


Assessing Your Current Observability Landscape
Let's go ahead and start by taking stock of where you are right now. It's like doing a health check-up for your observability setup. Here's what you need to do:
Audit Your Tools: Make a list of all the observability tools you're currently using. And I mean all of them - from the fancy APM solution to that homegrown script Bob in DevOps swears by.
Map Your Data Flow: Understand how data moves through your system. Where is it coming from? Where is it going? Are there any bottlenecks?
Identify Gaps and Redundancies: Are you missing visibility in critical areas? Are you collecting the same data in multiple places?
Assess Costs: This isn't just about license fees—factor in storage costs, personnel time, and any hidden expenses.
This audit might be a bit of an eye-opener. I've seen companies realise they're paying for tools they barely use or have massive blind spots in critical systems. But don't worry; knowledge is power, and this is the first step to optimisation.
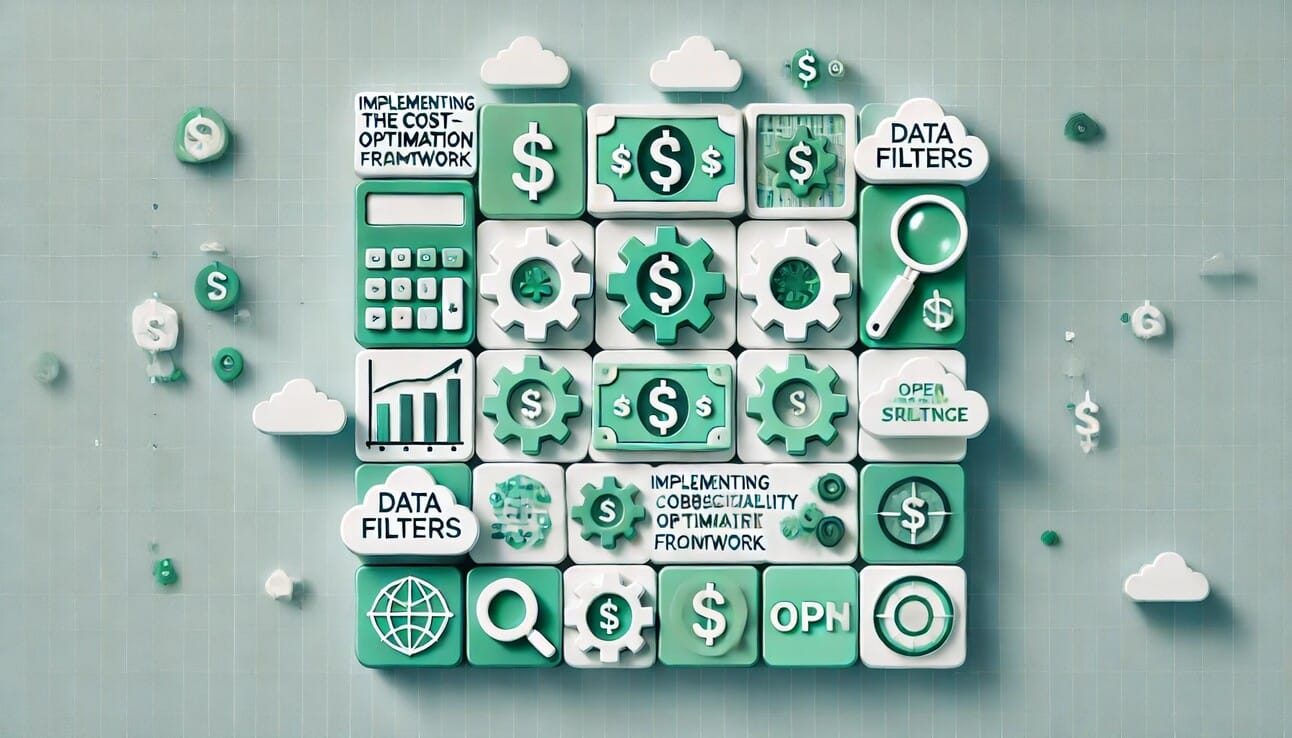
Implementing the Cost-Optimization Framework
Remember that 20-30% rule we discussed in our earlier article? That's your North Star here. Aim to keep your Observability costs between 20% and 30% of your total infrastructure spend. It's not a hard and fast rule, but it's a good benchmark to aim for.
Now, let's revisit some key strategies we've discussed:
Data Filtering: Not all data is created equal. Be ruthless about filtering out low-value data. It's like decluttering your house, keeping what's practical and tossing what's not. The image of data being filtered through a funnel perfectly captures this concept.
Open-Source Solutions: Don't overlook the power of open-source tools. They can offer robust capabilities at a fraction of the cost of commercial solutions. But remember, "free" doesn't always mean "no cost" - factor in the time and expertise needed to implement and maintain these tools.
AI and Machine Learning: These aren't just buzzwords. When implemented correctly, AI and ML can significantly reduce the manual work involved in observability, freeing up your team for more strategic tasks.
Usage-Based Pricing: If you're still on a fixed-price model, it might be time to renegotiate. Usage-based pricing can often lead to significant savings, especially if your usage fluctuates.

Optimising Data Collection and Storage
Here's where we get into the nitty-gritty. Effective data management is key to a cost-effective observability strategy. Let's break it down:
Innovative Sampling: You don't need to collect every single data point. Use intelligent sampling techniques to reduce data volume without losing essential insights.
Data Lifecycle Management: Not all data needs to be kept forever. Implement policies to archive or delete data based on its age and relevance.
Compression Techniques: Use data compression to reduce storage costs. However, don't sacrifice query performance in the process.
Remember, the goal isn't just to collect less data - it's to collect the right data. It's about quality over quantity.

Enhancing Observability with AI and Machine Learning
I know, I know - AI and ML are the buzzwords du jour. But hear me out. When used correctly, these technologies can be game-changers for your observability strategy.
Here's how:
Anomaly Detection: AI can spot patterns and anomalies much faster than humans, helping you catch issues before they become critical.
Predictive Analytics: Imagine predicting and preventing outages before they happen. That's the power of ML-driven predictive analytics.
Automated Root Cause Analysis: AI can help you pinpoint the root cause of issues faster, reducing mean time to resolution (MTTR).
But let's keep it real - AI and ML aren't magic bullets. They require good data, careful implementation, and ongoing maintenance. Don't just jump on the AI bandwagon because it's trendy. Make sure it's the right fit for your needs.

Aligning Observability with Business Objectives
Here's a truth bomb: if your observability strategy isn't aligned with your business objectives, you're doing it wrong. It's not just about collecting data or monitoring systems - it's about providing insights that drive business value.
Here's how to make that alignment happen:
Map Metrics to KPIs: Every metric you track should tie back to a business KPI. Suppose it doesn't ask yourself why you're tracking it.
Speak the Language of Business: Translate technical metrics into business impact when reporting on observability. Don't talk about CPU utilization - talk about how system performance is affecting customer satisfaction or revenue.
Demonstrate ROI: Be prepared to show how your observability strategy contributes to the bottom line. This might mean tracking metrics like reduced MTTR, improved customer satisfaction, or increased uptime.
Implementing a cost-effective observability strategy isn't just about tools and processes - it's about people and culture

Building a Culture of Observability
Implementing a cost-effective observability strategy isn't just about tools and processes - it's about people and culture. Here's how to foster a culture of observability in your organization:
Education and Training: Invest in training your team on observability best practices. Ensure everyone understands the importance of observability and how to use the tools effectively.
Cross-functional collaboration: Encourage collaboration between development, operations, and business teams. Observability isn't just an ops concern - it's everyone's responsibility.
Continuous Improvement: Implement regular reviews and retrospectives to identify areas for improvement in your observability practices.
Lead by Example: Leadership should champion the importance of observability and demonstrate its value to the organization.
Implementing a comprehensive Observability strategy isn't all sunshine and rainbows. Here are some common challenges you might face and how to overcome them:
Data Overload: The sheer volume of data can easily overwhelm you. Focus on collecting actionable data and use filtering and sampling techniques to manage the volume.
Tool Sprawl: As your observability needs to grow, you might find yourself with an assortment of tools. Regularly review your toolset and look for opportunities to consolidate.
Skills Gap: Observability requires a specific skill set. Invest in training your team, or consider hiring experts.
Resistance to Change: Implementing new observability practices might be challenging. To overcome this, focus on demonstrating value and involving team members.

Future-Proofing Your Observability Strategy
The only constant in tech is change, right? So, how do you build an observability strategy that can stand the test of time? Here are some tips:
Stay Flexible: Don't lock yourself into a single vendor or technology. Keep your options open and be prepared to adapt.
Invest in Skills: Technology changes, but the fundamental principles of observability remain. Invest in building your team's skills in data analysis, system architecture, and performance optimization.
Embrace Open Standards: Technologies like OpenTelemetry are gaining traction for a reason. They offer flexibility and future-proofing that proprietary solutions can't match.
Keep Learning: Stay up-to-date with the latest trends and technologies in observability. But don't just chase the shiny new thing - evaluate new technologies based on their potential to solve real problems for your organization.
Sponsor
Growth School is very grateful for sponsoring this series. If you would like to work with us, contact me directly, and let’s see how we can help each other.
💥 Use AI to 10X your productivity & efficiency at work (free bonus) 🤯
Still struggling to achieve work-life balance and manage your time efficiently?
Join this 3 hour Intensive Workshop on AI & ChatGPT tools (usually $399) but FREE for first 100 readers.
Save your free spot here (seats are filling fast!) ⏰
An AI-powered professional will earn 10x more. 💰 An AI-powered founder will build & scale his company 10x faster 🚀 An AI-first company will grow 50x more! 📊 |
Want to be one of these people & be a smart worker?
Free up 3 hours of your time to learn AI strategies & hacks that less than 1% people know!
🗓️ Tomorrow | ⏱️ 10 AM EST
In this workshop, you will learn how to:
✅ Make smarter decisions based on data in seconds using AI
✅ Automate daily tasks and increase productivity & creativity
✅ Skyrocket your business growth by leveraging the power of AI
✅ Save 1000s of dollars by using ChatGPT to simplify complex problems

Continuous Improvement and Feedback Loops
Remember, implementing a cost-effective observability strategy isn't a one-and-done deal. It's an ongoing process that requires continuous improvement and feedback. Here's how to keep your strategy evolving:
Regular Reviews: Schedule quarterly reviews of your observability strategy. This will allow you to assess what's working, what's not, and where you can improve.
Feedback Mechanisms: Set up channels for your team to provide feedback on the observability tools and processes. They're on the front lines - their insights are invaluable.
Stay Informed: Monitor industry trends and new technologies. Attend conferences, read blogs, and network with other professionals.
Experiment: Don't be afraid to try new things. Set up small pilot projects to test new tools or approaches before rolling them out widely.

Case Study: Putting Theory into Practice
Let's bring this down to earth with a real-world example. I recently worked with a mid-sized e-commerce company, which is called “TechCompanyA” (got to love an NDA), struggling with skyrocketing observability costs. They were spending over 50% of their infrastructure budget on Observability tools, yet they were still missing critical issues that impacted customer experience and revenue.
Initial Assessment:
Infrastructure: Multi-cloud environment using AWS and Google Cloud Platform
Annual Revenue: $50 million
Monthly Infrastructure Costs: $200,000
Monthly Observability Costs: $110,000 (55% of infrastructure budget)
Tech Stack: Microservices architecture with 50+ services
Existing Tools: New Relic, Datadog, ELK Stack, custom in-house solutions
Here's a detailed breakdown of the steps we took:
Audit and Consolidation:
We conducted a comprehensive audit of their Observability stack and found they used multiple tools with overlapping functionality. This led to data duplication and increased costs.
Actions taken, Recommendation given:
Mapped all services to their corresponding observability tools
Identified redundancies in log collection, metrics monitoring, and tracing
Consolidated to a core set of tools:
Metrics: Prometheus
Logging: ELK Stack (optimized)
Tracing: Jaeger
Visualization: Grafana
Data Optimization
TechCompanyA collected and stored vast amounts of data, rarely accessed or provided little value.
Actions taken:
Implemented clever sampling techniques:
Probabilistic sampling for high-volume, low-value logs
Reservoir sampling for maintaining representative data sets
Established data lifecycle policies:
Hot storage (1 week): High-resolution metrics and logs
Warm storage (1 month): Aggregated metrics and filtered logs
Cold storage (3 months+): Highly aggregated data for long-term trend analysis
Optimized log levels:
Reduced DEBUG level logging in production
Implemented dynamic log levels for troubleshooting
Result: 40% reduction in data volume, leading to significant storage cost savings
Open Source Integration
We replaced several proprietary tools with open-source alternatives, focusing on core observability needs.
Actions taken:
Implemented Prometheus for metrics collection and alerting
Deployed Grafana for visualization
Optimized ELK Stack for log management
Implemented OpenTelemetry for distributed tracing:
Instrumented essential services with OpenTelemetry SDKs
Set up OpenTelemetry Collector for data processing and export
Integrated with Jaeger for trace visualization and analysis
Configured end-to-end tracing across microservices:
Implemented context propagation between services
Added custom attributes to traces for business-specific insights
Set up trace sampling strategies to balance data volume and insights
Result: Improved visibility into service dependencies and performance bottlenecks, leading to faster issue resolution and better system optimization
Open source isn't truly 'free': While open source tools eliminated some licensing costs, we had to account for increased expenses in training, support, and infrastructure. A clear strategy and gradual ramp-up were essential to manage these costs effectively.
AI-Driven Anomaly Detection:
We implemented an AI-driven anomaly detection system to address the issue of missing critical problems.
Actions taken:
Deployed an open-source anomaly detection tool (Skyline)
Integrated with existing metrics from Prometheus
Trained the model on historical data to establish baselines
Implemented adaptive thresholds to reduce false positives
Set up automated alerts for detected anomalies
Result: Caught several critical issues before they impacted customers, including:
A slow-growing memory leak in a vital microservice
Unusual patterns in order processing times during peak hours
Intermittent network latency issues affecting a subset of users
Personnel Training and C-Level Education
We implemented a comprehensive training program to ensure the success of the new observability strategy.
Actions taken:
Conducted hands-on workshops for engineering teams:
OpenTelemetry instrumentation best practices
Effective use of Prometheus and Grafana
Advanced log analysis techniques
Organized "Lunch and Learn" sessions on observability concepts
Developed an internal knowledge base for observability best practices
Created a mentorship program pairing observability experts with junior team members
For C-level executives:
Conducted an "Observability for Executives" workshop
Developed custom dashboards showing key business metrics derived from observability data
Created monthly reports translating technical improvements into business impact
Result: Increased team efficiency in using observability tools, better alignment between technical and business goals, and more vital executive support for observability initiatives.
C-Level Support and Resource Allocation
A critical factor in the success of this project was the unwavering support and trust from C-level executives. They understood that achieving significant improvements in just six months required:
Dedicated resources: A team of skilled engineers was assigned full-time to the project.
Training budget: Funds were allocated to upskill the team in new tools and practices.
Long-term commitment: Leadership agreed to a multi-year strategy for continuous improvement in observability practices.
This level of support was crucial in overcoming initial hurdles and maintaining momentum throughout the project.
Skill Development and Long-Term Planning
We recognized that transitioning to a new observability stack required both immediate action and long-term planning:
Hired two observability specialists to lead the transition and train existing staff.
Developed a 2-year roadmap for continuous skill development in the team.
Established partnerships with local universities to create an observability internship program, ensuring a pipeline of skilled talent.
Vendor Contract Review and Cost Analysis
While moving to open-source tools provided significant savings, we carefully considered the total cost of ownership:
Reviewed existing vendor contracts to understand termination clauses and potential penalties.
Factored in the cost of enterprise support for open-source tools (e.g., Grafana Enterprise).
Considered the increased personnel costs for managing and maintaining open-source solutions.
After thorough analysis, the projected savings were adjusted to account for these factors:
Initial projected savings: 54% reduction in monthly observability costs
Adjusted savings after factoring in new expenses: 40% reduction
It's important to note that the cost savings were tied to terminating off-the-shelf vendor contracts. We negotiated a phased exit to minimize disruption and avoid penalties.
The Final Outcome
Within six months, TechCompanyA had reduced their observability spend to 30% of their infrastructure budget ($60,000/month), while significantly improving their ability to detect and resolve issues. Key improvements included:
40% reduction in monthly observability costs (adjusted from the initial 54% projection)
30% decrease in Mean Time to Detection (MTTR) for critical issues
40% improvement in Mean Time to Resolution (MTTR)
99.99% uptime achieved, up from 99.9%
25% reduction in customer-reported issues due to improved proactive problem detection
15% increase in development team productivity due to better observability practices
The implementation of OpenTelemetry and distributed tracing provided several key benefits:
Identified and resolved a complex race condition in the order processing pipeline, improving order completion rates by 5%
Pinpointed database query optimizations that reduced average response times by 200ms
Discovered and fixed inefficient API calls between microservices, reducing overall system load by 10%
Lessons Learned:
C-level support is crucial for rapid, significant changes in observability practices.
The right resources and skills are both an initial and long-term commitment.
When transitioning to open-source tools, factor in all costs, including potential licensing for enterprise support and increased personnel costs.
Cost savings from vendor transitions may take time to realize due to contract obligations fully.
A holistic approach yields the best results by combining tool optimization, personnel training, and process improvements.
This case study demonstrates that with a strategic approach to observability, including open-source tools, comprehensive training, and executive buy-in, companies can significantly reduce costs while improving their ability to monitor and maintain complex systems effectively. However, it also highlights the importance of thorough planning, resource allocation, and realistic cost projections when undertaking such a transformation.
Wrapping Up: Your Next Steps in Cost-Effective Observability
I hope you're excited and equipped to tackle your observability challenges as we wrap up this deep dive into building a comprehensive, cost-effective observability strategy. This series has covered a lot of ground, from understanding the cost conundrum to leveraging open-source tools and creating a comprehensive approach.
The future of observability is bright, but it's also complex. As systems become more distributed and ephemeral, the challenges of gaining visibility will only grow. But so will the opportunities. With the right strategy, tools, and mindset, you can turn observability from a cost centre into a competitive advantage.
Remember, there's no one-size-fits-all solution. What works for a Silicon Valley unicorn might not work for your enterprise. The key is understanding your unique needs, aligning your strategy with your business objectives, and adapting as technology and best practices evolve.
Now, this seems like a lot to take on. You might be thinking, "Where do I even start?" or "Do I have the resources to implement all of this?" That's where I come in. I'm not just here to share information – I'm here to help you put it into practice.
If you're overwhelmed or want expert guidance, I'd happily work with you directly. Whether it's conducting an initial audit, helping you benchmark your costs, or developing a tailored strategy for your organisation, I'm here to help you navigate this complex landscape.
So, what's your next step? It could be conducting that observability audit we talked about, exploring how AI could enhance your current setup, or reaching out to me for a personalised consultation. Whatever it is, I encourage you to take action. The world of Observability is moving fast, and you want to be included.
Thanks for joining me through the "Beyond the Numbers" series. Here's to building more observable, reliable, and cost-effective systems. Until next time, keep observing and optimising. Remember, I'm just a message away if you need hands-on support in transforming your observability strategy.
Reply